In the digital age, data has become the lifeblood of organizations across industries. From understanding customer behavior to optimizing operations, the ability to harness and analyze data effectively can spell the difference between success and stagnation.
With the advent of Artificial Intelligence (AI), the landscape of data analytics has undergone a profound transformation, offering unprecedented insights and opportunities. In this comprehensive guide, we delve into the intricacies of AI data analytics, exploring its intersection with cloud-based data management, synchronization of data systems, processing techniques, integration methods, visualization, and extraction.
Understanding AI Data Analytics
AI-driven data analytics is a dynamic field that encompasses the use of advanced algorithms and machine learning techniques to analyze vast volumes of data and derive actionable insights.
Unlike traditional analytics approaches, AI empowers organizations to uncover hidden patterns, predict future trends, and automate decision-making processes. Whether it's optimizing supply chains, personalizing customer experiences, or enhancing cybersecurity, AI data analytics has emerged as a game-changer across diverse domains.
Cloud-Based Data Management
In the era of big data, managing and storing vast datasets efficiently is a paramount challenge for organizations. Cloud-based data management solutions offer a scalable and flexible approach to address these challenges. By leveraging cloud infrastructure, businesses can access on-demand computing resources, streamline data storage, and ensure high availability and reliability.
Moreover, cloud platforms provide advanced analytics tools and services that seamlessly integrate with AI algorithms, enabling organizations to derive actionable insights in real-time.
Synchronizing Data Systems
In today's interconnected world, organizations operate across multiple platforms and systems, leading to fragmented data silos. Synchronizing data systems is crucial to ensure consistency, accuracy, and accessibility of data across the enterprise. Through the adoption of robust data integration strategies and technologies, organizations can break down silos, harmonize disparate datasets, and establish a single source of truth.
- Extract, Transform, Load (ETL) Processes: ETL processes involve extracting data from various sources, transforming it into a standardized format, and loading it into a target system. This approach ensures data consistency and integrity across different platforms.
- Data Virtualization: Data virtualization allows organizations to access and manipulate data from disparate sources without physically moving or replicating it. By creating virtualized views of data, organizations can simplify data access and accelerate decision-making processes.
- API-Based Integration: Application Programming Interface (API)-based integration enables seamless communication between different software applications. By exposing data through APIs, organizations can facilitate real-time data exchange and integration, enabling agile decision-making.
Data Processing Techniques
AI-driven data processing techniques have revolutionized the way organizations extract insights from their data assets. From natural language processing (NLP) to image recognition and predictive analytics, AI algorithms are capable of processing diverse data types at scale.
Deep Learning Algorithms
Deep learning algorithms, such as neural networks, mimic the structure and function of the human brain, enabling them to learn from vast amounts of data. These algorithms excel in handling unstructured data such as images, videos, and text, making them ideal for tasks such as image recognition, sentiment analysis, and language translation.
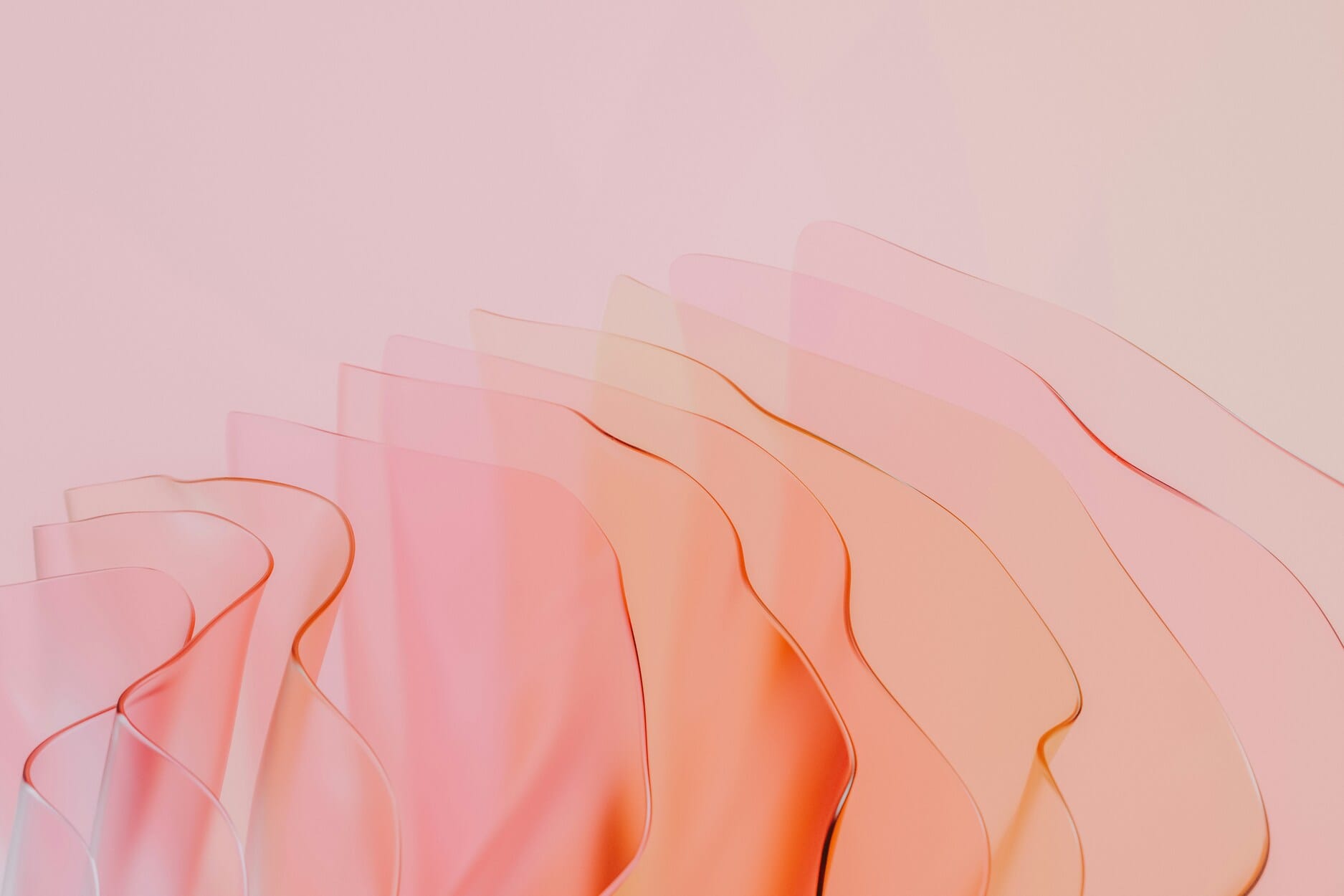
Reinforcement Learning:
Reinforcement learning is a type of machine learning where an agent learns to make decisions by interacting with its environment. This approach has applications in fields such as robotics, gaming, and finance, where agents must learn optimal strategies through trial and error.
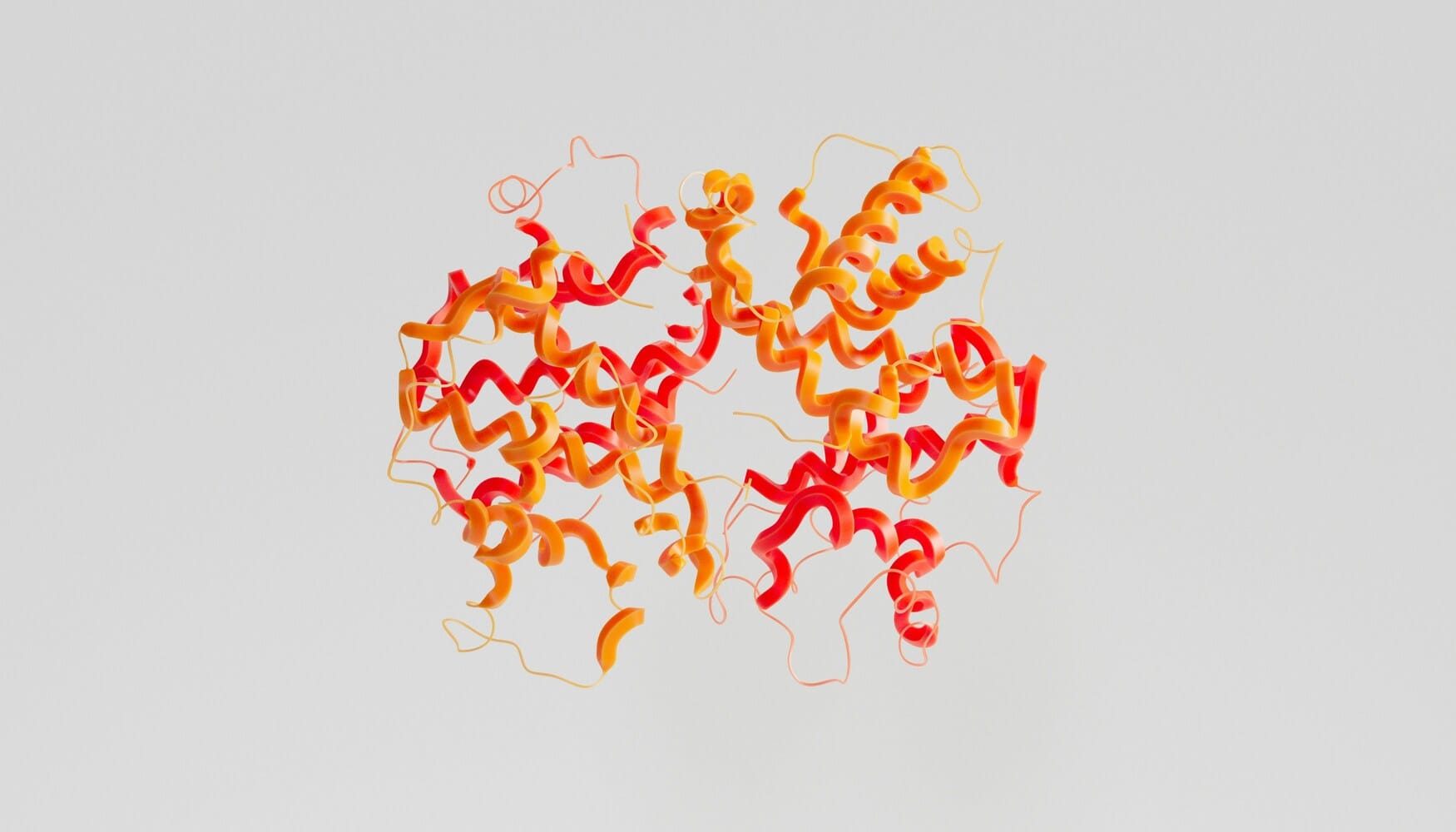
Transfer Learning:
Transfer learning involves leveraging knowledge from one domain to improve performance in another domain. By pre-training models on large datasets, organizations can accelerate the learning process and achieve better results with limited data.
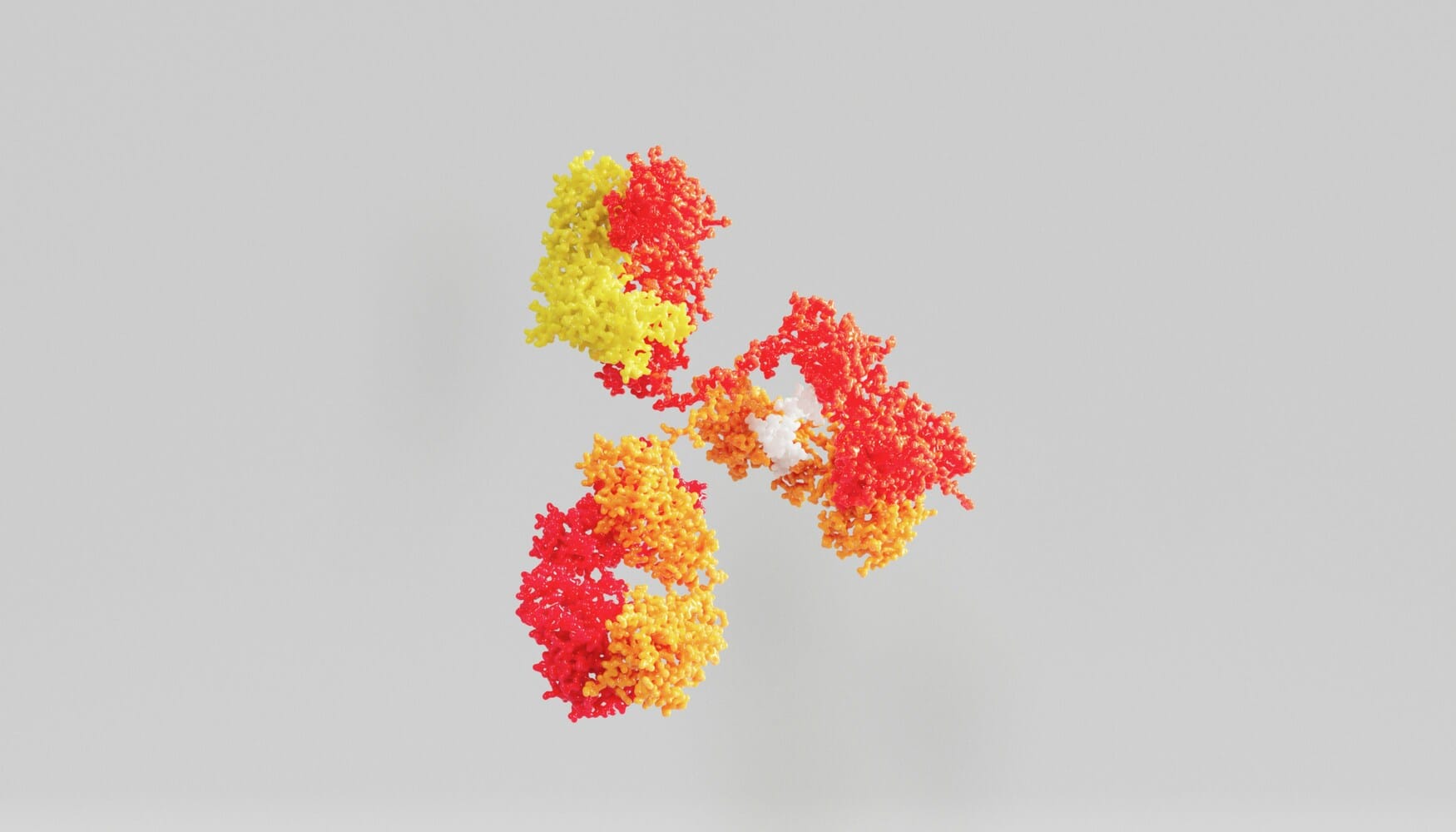
Facilitating Data Integration
Data integration lies at the heart of effective data analytics initiatives, facilitating the aggregation of disparate datasets for holistic insights. Advanced data integration platforms leverage AI and machine learning algorithms to automate the process of data mapping, cleansing, and transformation.
Batch processing involves processing data in predefined batches, while real-time data streaming enables continuous processing of data as it arrives. Depending on the use case, organizations may opt for batch processing for historical analysis or real-time streaming for timely insights.
The Power of Data Visualization
Data visualization plays a pivotal role in democratizing data insights and enabling stakeholders to make informed decisions. Interactive dashboards, charts, and graphs transform raw data into visually compelling narratives, making complex information accessible and actionable.
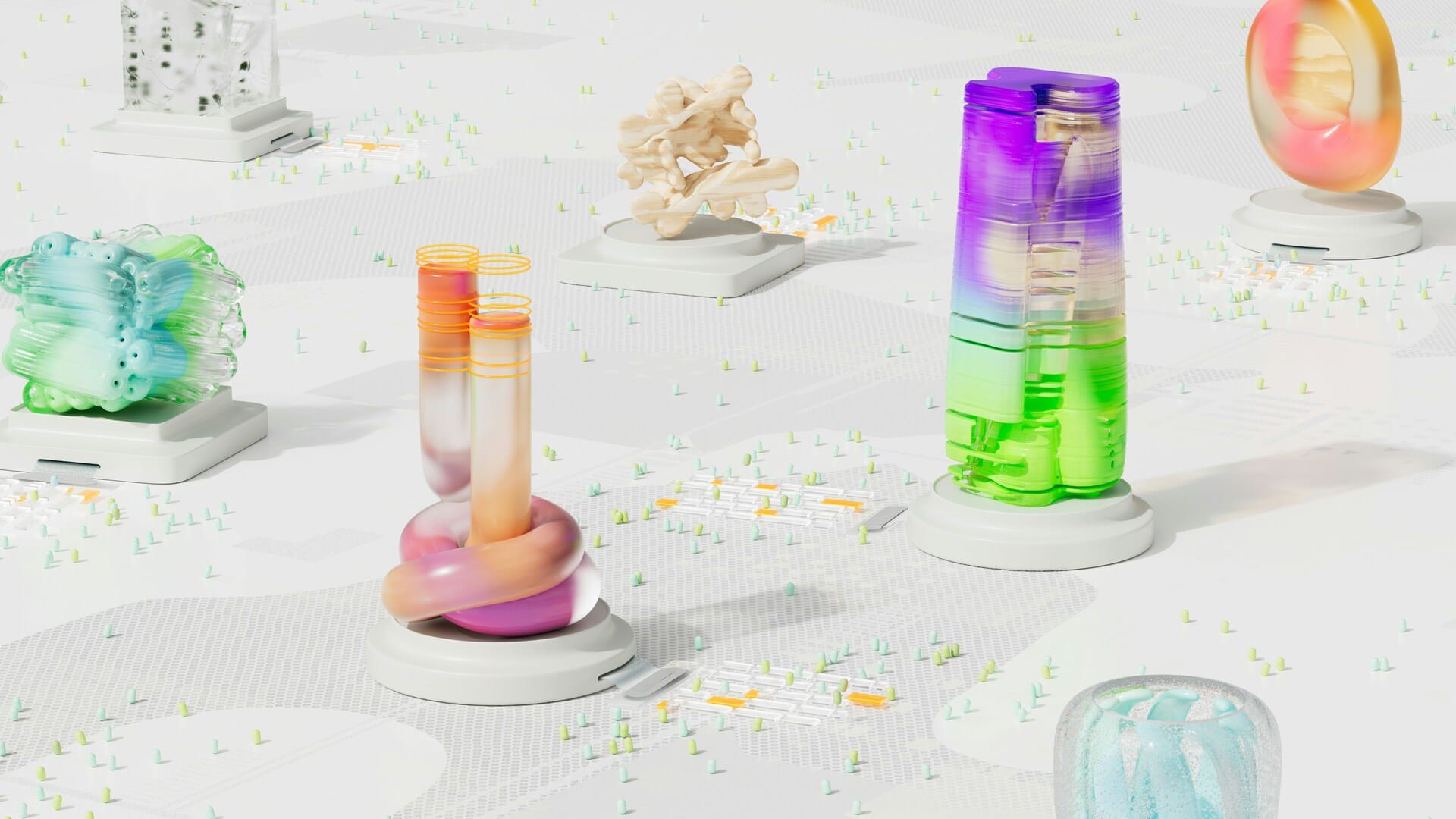
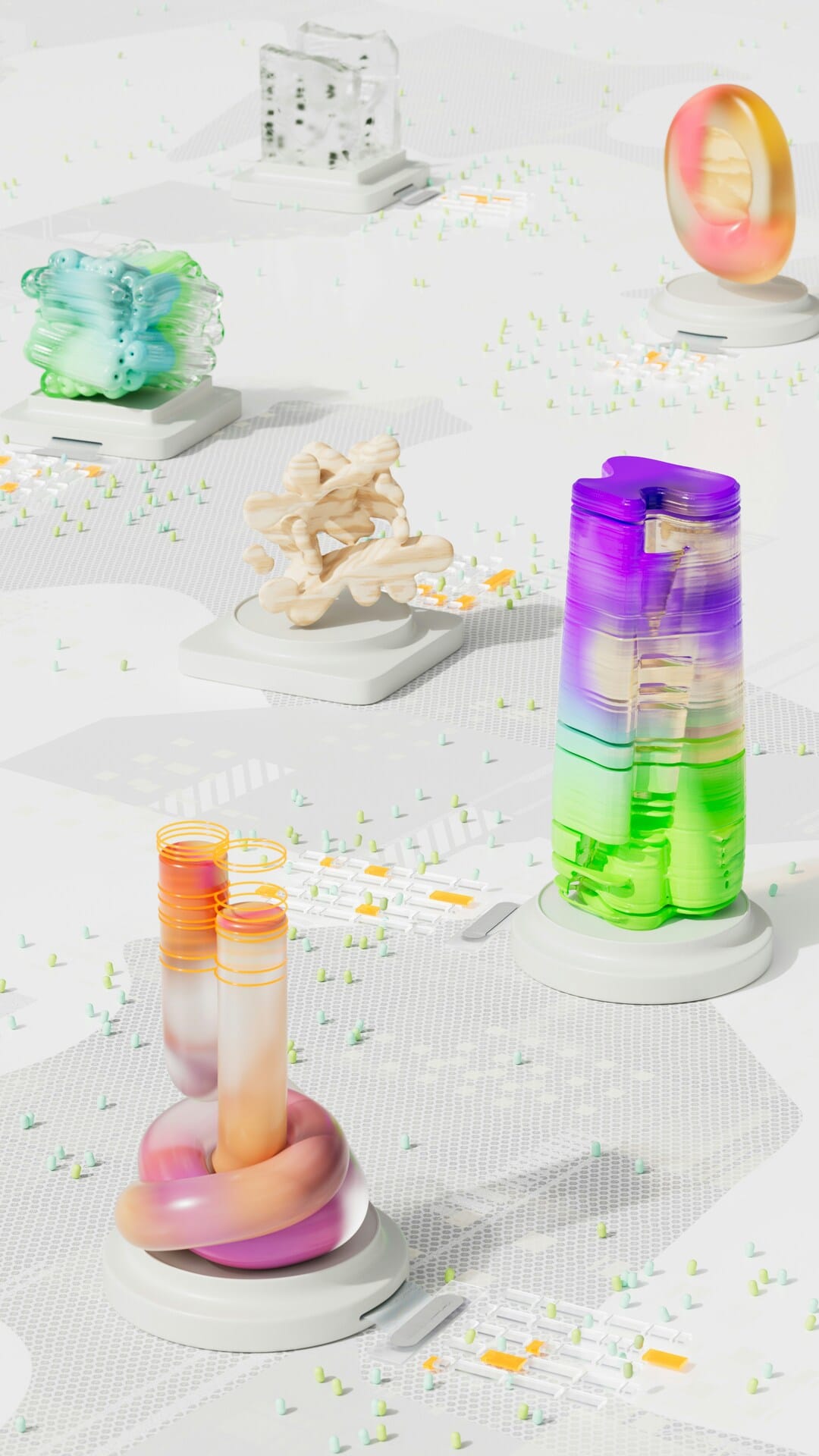
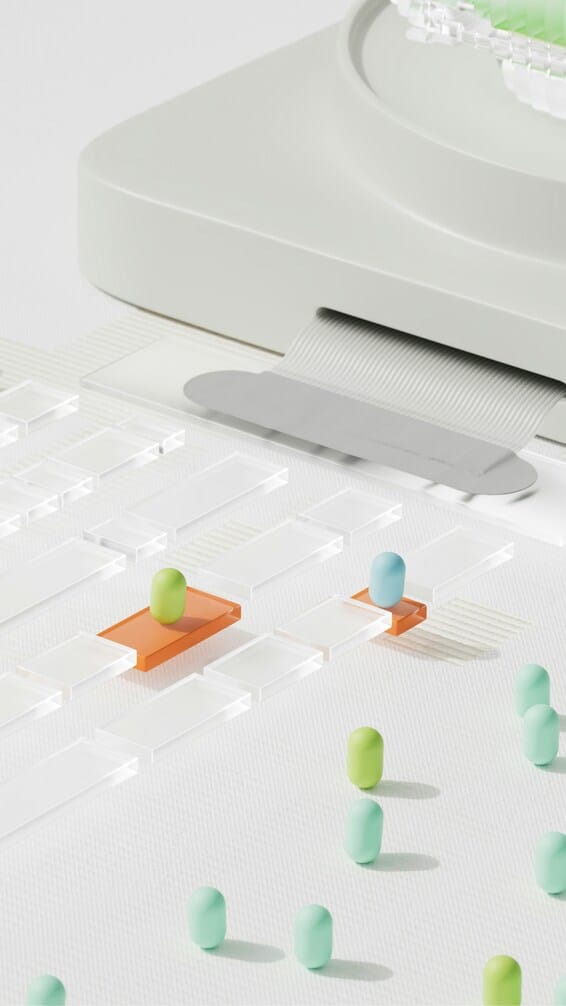
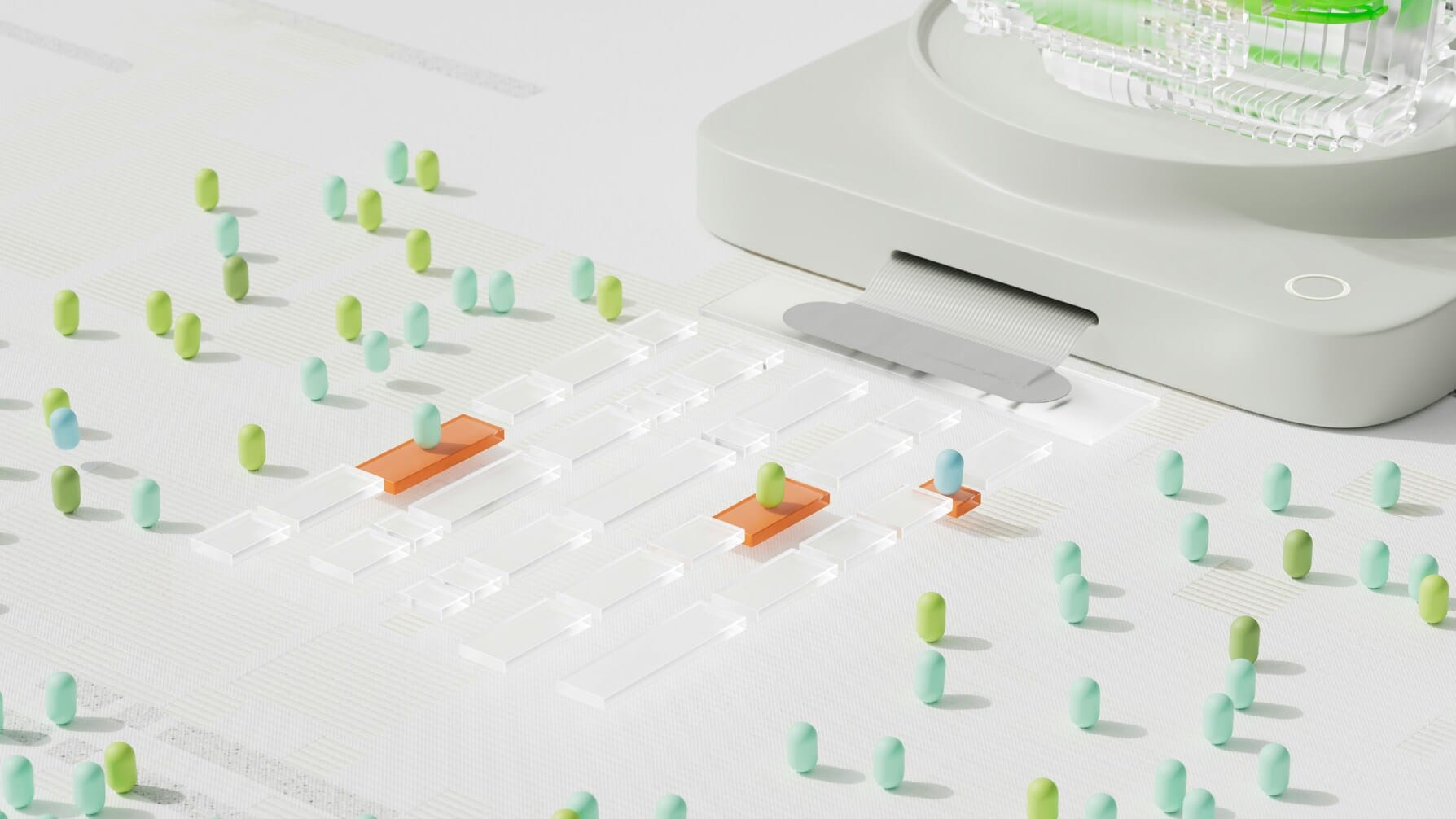
Data visualization
Interactive Dashboards
Interactive dashboards allow users to explore data dynamically, drill down into details, and uncover insights through interactive visualizations. By customizing dashboards to suit specific user needs, organizations can empower stakeholders at all levels to make data-driven decisions.
Geospatial Visualization
Geospatial visualization techniques enable organizations to analyze and visualize data in geographic contexts. Whether it's plotting customer locations on a map or visualizing weather patterns, geospatial visualization provides valuable insights into spatial relationships and trends.
Temporal Visualization
Temporal visualization techniques help organizations analyze data over time, identify trends, and forecast future developments. Whether it's tracking sales trends, monitoring stock prices, or predicting demand, temporal visualization enables organizations to make timely decisions based on historical data patterns.
Streamlining Data Extraction Processes
Efficient data extraction is fundamental to AI-driven analytics initiatives, enabling organizations to unlock the value of their data assets. Automated data extraction techniques leverage AI algorithms to parse through unstructured data sources such as documents, emails, and web pages.
- Natural Language Processing (NLP): NLP algorithms extract meaningful information from unstructured text data, such as emails, articles, and social media posts. By analyzing the context, syntax, and semantics of text, NLP algorithms can identify entities, relationships, and sentiments, enabling organizations to derive actionable insights from textual data.
- Optical Character Recognition (OCR): OCR techniques convert scanned documents, images, and PDFs into machine-readable text. By digitizing unstructured data, OCR enables organizations to analyze and process large volumes of documents quickly and accurately, reducing manual effort and accelerating decision-making processes.
- Web Scraping: Web scraping involves extracting data from websites and web pages using automated scripts or bots. Whether it's scraping product prices from e-commerce sites, gathering news articles from online sources, or monitoring social media mentions, web scraping provides organizations with valuable data for analysis and decision-making.
Conclusion
In conclusion, AI data analytics represents a paradigm shift in the way organizations harness the power of data to drive innovation and gain a competitive edge. By leveraging cloud-based data management, synchronizing data systems, adopting advanced processing techniques, integrating disparate datasets, visualizing insights, and automating data extraction, businesses can unlock the full potential of their data assets.
As AI continues to evolve and mature, organizations must embrace a data-driven culture and invest in the necessary infrastructure and talent to stay ahead of the curve. With AI as a catalyst, the possibilities for data-driven innovation are limitless.